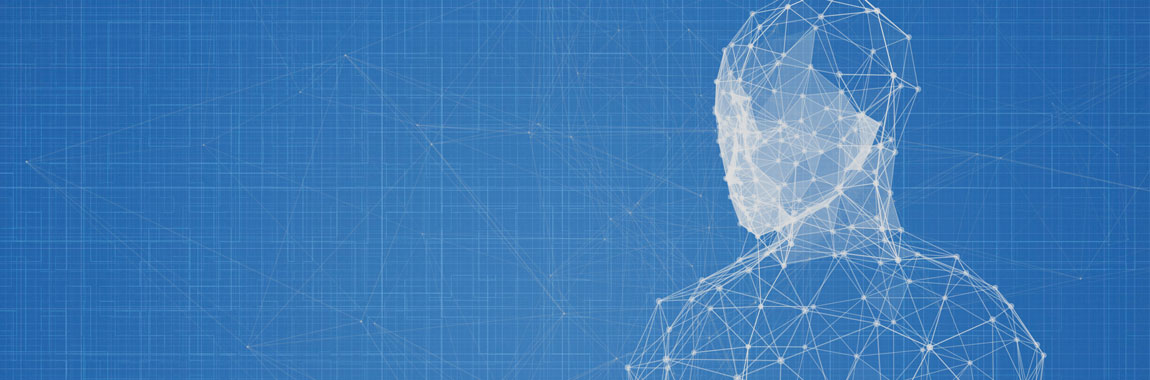
SMITH BRAIN TRUST – Attempting to conduct predictive modeling from sparse, binary data sets is complicated. So researchers typically use what’s called unsupervised matrix-factorization based dimensionality reduction as an initial step in the process. But it’s not clear whether dimensionality reduction actually helps improve predictive modeling performance.
Textbooks often recommend supervised regularization as a better alternative, though researchers and other practitioners tend to shun that recommendation, particularly when dealing with large, sparse feature sets.
In new research, Maryland Smith’s Jessica M. Clark conducts a series of experiments to gauge whether unsupervised dimensionality reductions improves the generalization performance of binary classifiers that use massive, sparse data sets.
It is believed to be the first study to comprehensively evaluate whether dimensionality reduction improves the predictive modeling performance amid state-of-the-art complexity control techniques. The study aims to lend insights to anyone who leverage predictive modeling for their research or work.
“Ultimately the core lesson of this paper,” Clark and co-author Foster Provost from NYU’s Stern School of Business write, “can be summarized as one of the basic system design principles: exercise caution when adding complexity via a dimensionality reduction step to the predictive modeling process, even if one feels confident that DR will benefit the performance.”
The principle is “frequently violated” in the predictive modeling literature, they write. And their comprehensive research reveals that “that this violation is a mistake that leads to weaker results than might otherwise be possible.”
Read more: “Unsupervised dimensionality reduction vs. supervised regularization for classification from sparse data,” by Jessica Clark and Foster Provost, Data Mining and Knowledge Discovery.
Jessica M. Clark is assistant professor of information systems at the University of Maryland's Robert H. Smith School of Business.
Research interests: Use of machine learning techniques and individual-level data to explore the relationship between demographic characteristics and behaviors, and how that relationship affects financial or social outcomes. Past work has included developing algorithms for disambiguating consumers’ use of a shared device (specifically, a television Set-Top Box); investigating the utility of highly fine-grained transactional data for predicting consumers’ responses to marketing offers at a bank; and evaluating the utility of commonly used statistical modeling techniques in the context of massive data sets. Her current interests focus on using analytics to better understand racial and gender dynamics on online platforms such as Kickstarter.com and Meetup.com.
Selected accomplishments: 2017 European Research Paper of the Year by the Association for Information Systems; member of a winning team at the first ever paper-a-thon at the International Conference on Information Systems in Seoul, Korea.
About this series: Maryland Smith celebrates Women Leading Research during Women’s History Month. The initiative is organized in partnership with ADVANCE, an initiative to transform the University of Maryland by investing in a culture of inclusive excellence. Other Women's History Month activities include the eighth annual Women Leading Women forum on March 5, 2019.
Other fearless ideas from: Rajshree Agarwal | Ritu Agarwal | T. Leigh Anenson | Kathryn M. Bartol | Christine Beckman | Margrét Bjarnadóttir | M. Cecilia Bustamante | Jessica M. Clark | Rellie Derfler-Rozin | Waverly Ding | Wedad J. Elmaghraby | Rosellina Ferraro | Rebecca Hann | Amna Kirmani | Hanna Lee | Hui Liao | Jennifer Carson Marr | Wendy W. Moe | Courtney Paulson | Louiqa Raschid | Rebecca Ratner | Rachelle Sampson | Debra L. Shapiro | M. Susan Taylor | Niratcha (Grace) Tungtisanont | Vijaya Venkataramani | Janet Wagner | Yajin Wang | Liu Yang | Jie Zhang | Lingling Zhang
GET SMITH BRAIN TRUST DELIVERED
TO YOUR INBOX EVERY WEEK
Media Contact
Greg Muraski
Media Relations Manager
301-405-5283
301-892-0973 Mobile
gmuraski@umd.edu
Get Smith Brain Trust Delivered To Your Inbox Every Week
Business moves fast in the 21st century. Stay one step ahead with bite-sized business insights from the Smith School's world-class faculty.