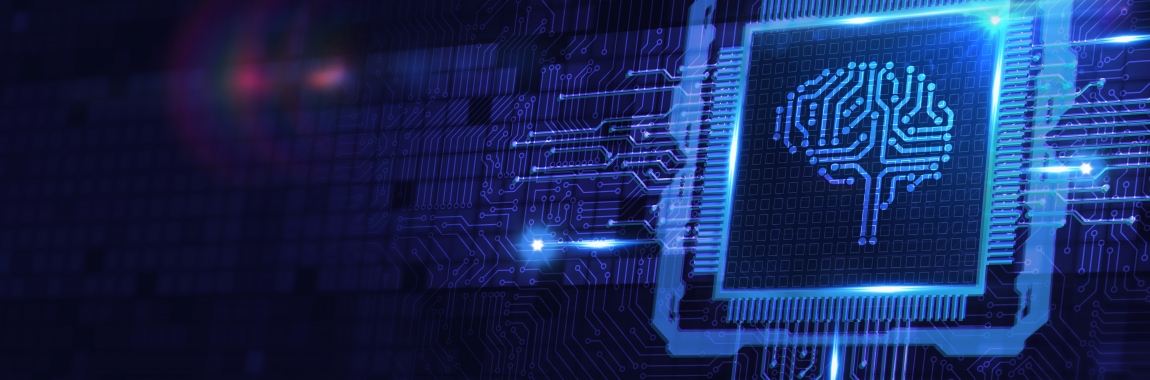
Application of artificial intelligence (AI) machine learning techniques in industry and government for data-driven predictive analytics has been based primarily on statistical econometric models. However, one of the limitations of this approach is the “black-box” nature of the resulting models, which frequently result in a lack of interpretability.
Michael C. Fu, the Smith Chair of Management Science and chair of the Decision, Operations and Information Technologies Department at the University of Maryland’s Robert H. Smith School of Business, has a new approach for building such data-driven predictive analytics models, which can be viewed as either an alternative or a complementary approach to the traditional AI machine learning approaches.
Fu, who has a joint appointment with the Institute for Systems Research and an affiliate appointment with the Department of Electrical and Computer Engineering, both in the A. James Clark School of Engineering, recently co-authored a research article with Peking University’s Yijie Peng, Columbia University’s Henry Lam, and Free University of Amsterdam’s Bernd Heidergott, in which the standard maximum likelihood estimation problem is cast in a new light.
“We propose a new method for estimating unknown parameters of a stochastic model without assuming an analytical likelihood function,” write the researchers. The paper is featured in the journal Operations Research.
The researchers focus on interpretable stochastic models, which are more like physics-based models than black box econometric models. Stochastic models forecast the probability of various outcomes under different conditions, using random variables, to predict outcomes while accounting for certain amounts of unpredictability or randomness. Companies use these models to improve their business practices and increase profitability. And in the financial services, analysts, planners and portfolio managers use this type of modeling to manage assets and liability and optimize portfolios.
Using Monte Carlo simulations of the stochastic models, the researchers devise a way to find the best estimates of the stochastic model parameters based on generated output data using maximum likelihood estimation, the most commonly used statistical technique for machine learning data-driven models. “We directly fit the underlying stochastic model to the output data, which opens the possibility of extending data-driven ideas to causal stochastic models,” they write. In other words, “we bring light to the black box [of machine learning models].”
Read more: “Maximum Likelihood Estimation By Monte Carlo Simulation: Towards Data-Driven Stochastic Modeling,” is featured in Operations Research.
Media Contact
Greg Muraski
Media Relations Manager
301-405-5283
301-892-0973 Mobile
gmuraski@umd.edu
Get Smith Brain Trust Delivered To Your Inbox Every Week
Business moves fast in the 21st century. Stay one step ahead with bite-sized business insights from the Smith School's world-class faculty.