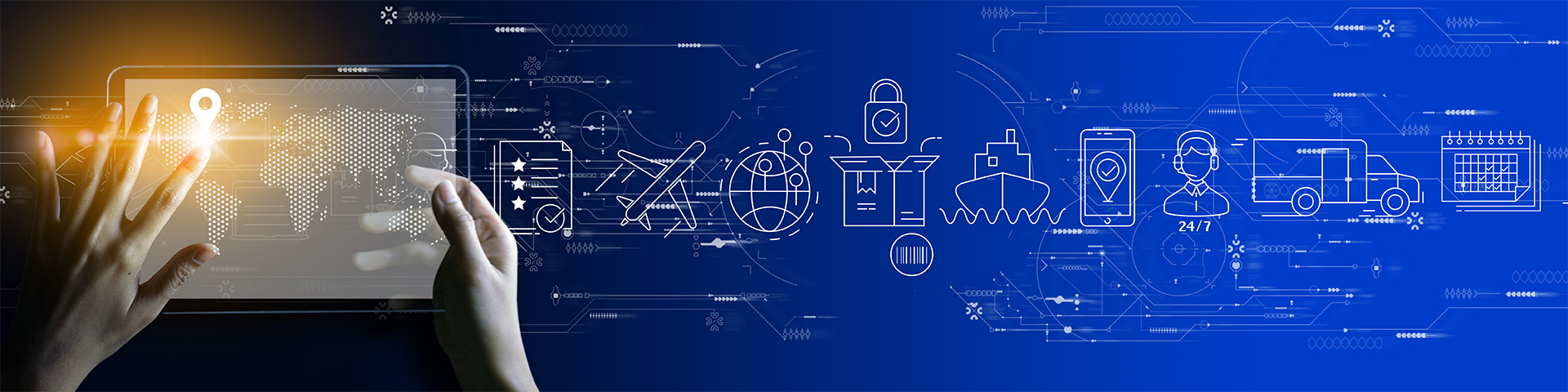
In a world of uncertainty, logistics firms must predict how many resources will be needed to achieve service objectives. According to the recent development of a statistical uncertainty model by Maryland Smith’s Ilya Ryzhov, predicting such costs is within reach.
Ryzhov, associate professor of Decision, Operations and Information Technology worked with colleagues from Maryland’s College of Civil and Environmental Engineering, as well as Virginia Commonwealth University and University of Utah. Their work, published in the journal Operations Research, considers situations where a central authority, like corporate headquarters, divides a resource between entities such as branch offices. The entities then autonomously use the resource to achieve their own local objectives, incurring costs for the firm.
“In a large city, it is difficult for a single decision-maker to coordinate an entire fleet of vehicles,” said Ryzhov. “A logistics firm could divide the city into geographical regions, divide its fleet between these regions and allow each region to coordinate its own portion of the fleet independent of other regions.”
Each region uses its resources to the best of its ability, however, due to day-to-day changes in demand, the cost is highly uncertain, which is where Ryzhov’s work comes in.
“The central authority likely has access to data that can help to estimate the cost for different resource levels,” Ryzhov said. “However, such data may be scarce or expensive to collect; furthermore, calculating costs from demand data can potentially be very computationally intensive.”
Using their newly developed statistical uncertainty model, Ryzhov and his team were able to reduce uncertainty by taking into account the decreasing trend of costs – more resources leads to lower costs.
“Our ‘robust’ decisions perform well in the sense of reducing the risk of worst-case outcomes,” Ryzhov said. “Demand often fluctuates and any region can experience sudden demand spikes. When this happens, regions that do not have enough resources will incur extremely high costs.”
Due to the ability of the model to effectively synergize with “robust optimization,” a decision-making methodology designed for small-data situations, the ‘robust’ resource allocation will perform better in ‘worst-case’ demand scenarios than standard resource allocation models. This has the potential to allow logistics firms everywhere to effectively plan costs and save for the future.
The research “Data Driven Robust Resource Allocation With Monotonic Cost Functions” was published in the journal Operations Research.
Media Contact
Greg Muraski
Media Relations Manager
301-405-5283
301-892-0973 Mobile
gmuraski@umd.edu
Get Smith Brain Trust Delivered To Your Inbox Every Week
Business moves fast in the 21st century. Stay one step ahead with bite-sized business insights from the Smith School's world-class faculty.