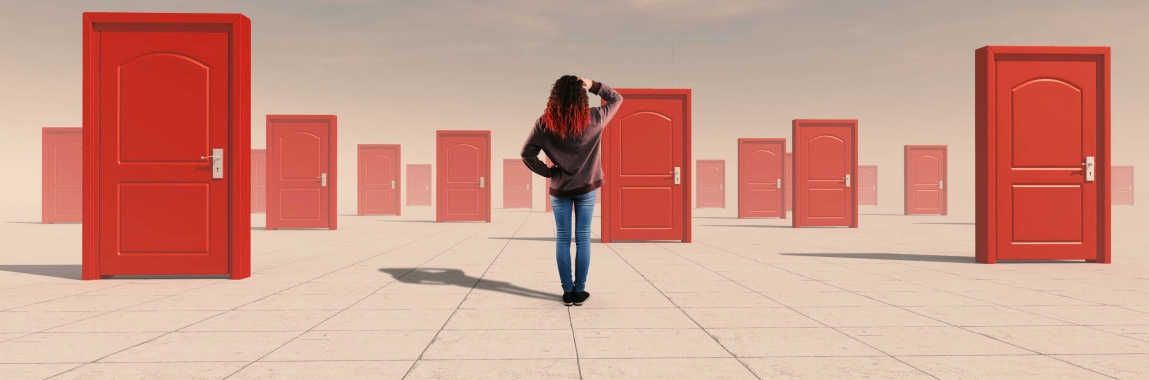
How do you make good decisions when you don’t have all the information in front of you? That’s the question Maryland Smith’s Ilya O. Ryzhov answers in new research published in the journal Operations Research.
Ryzhov, an associate professor of management science and operations management, worked with Ye Chen of Virginia Commonwealth University. They lay out a new framework to prove that it is possible to learn from indirect or incomplete information. Their approach applies a well-established statistical theory to use sequential learning to create a new way to incorporate incomplete data and improve the consistency of information for better decision-making.
“The main issue is that, in the problems we study, decision-makers are not able to directly observe the quantity that is most important for making decisions,” Ryzhov says.
Ryzhov offers several examples:
In pricing, a seller wants to know how much the buyer is willing to pay, but the buyer does not reveal this information. Instead, the seller quotes a price and the buyer either accepts or rejects that price. But if, for example, the price is accepted, the seller is left wondering if a slightly higher price would also have worked.
In medical trials, a patient receives a dose of a drug. If there are side effects, this may mean that the dose was too high. But it is not clear how much lower the dose should have been, only that it should have been lower.
In financial markets, one can encourage traders to buy and sell by setting bid and ask prices for a commodity. But, just like in pricing, one can only see if the current prices are too high/low. One does not directly see what the 'right' prices should be.
It’s a phenomenon known as “censored information” – where “we only learn indirectly about the important quantity,” says Ryzhov. “Many researchers and practitioners have developed various workarounds that allow them to learn from censored information.”
He points to one noteworthy success: Microsoft in the mid-2000s, where they used the win/loss patterns of users on their competitive gaming platform to learn the users' skill levels and improve how users were matched against each other. Many other methods of this type also worked well, he says. But, until now, there was no unifying scientific explanation of why these methods worked.
“This is what our paper explains,” says Ryzhov. “We create a mathematical framework that can be used to analyze all of these various settings and prove that learning works even with censored information. In other words, given enough time, indirect learning is still able to recover the important quantity even without direct observations of it.”
Read the full paper, “Technical Note — Consistency Analysis of Sequential Learning Under Approximate Bayesian Inference,” in Operations Research.
Media Contact
Greg Muraski
Media Relations Manager
301-405-5283
301-892-0973 Mobile
gmuraski@umd.edu
Get Smith Brain Trust Delivered To Your Inbox Every Week
Business moves fast in the 21st century. Stay one step ahead with bite-sized business insights from the Smith School's world-class faculty.