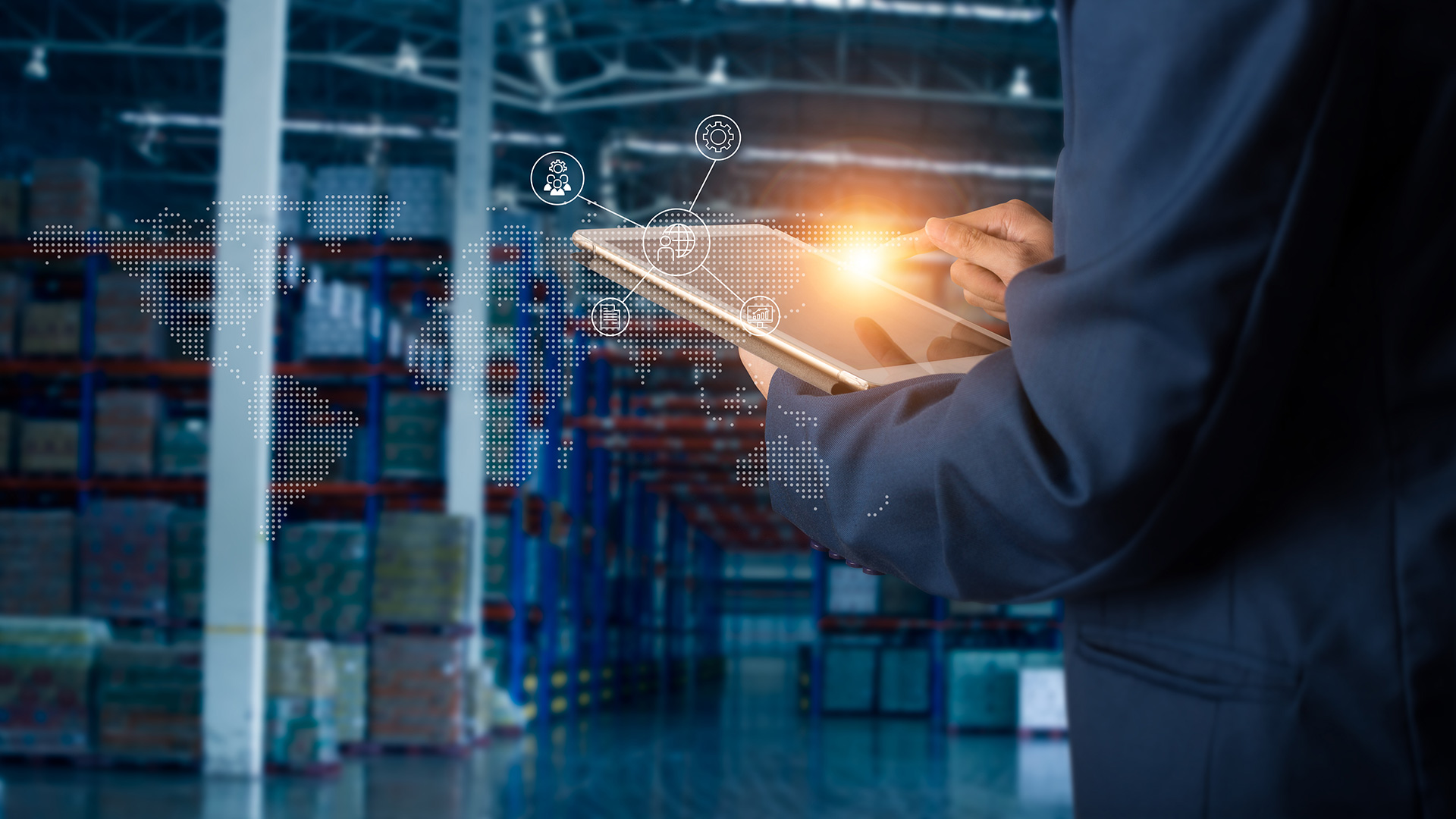
Planning capacity, inventory, marketing and financials for new products are explored in a new study from Smith.
As firms try to accurately forecast operational decisions, new research from the Robert H. Smith School of Business, inspired by Bayesian model averaging, looks at a “robust, and interpretable approach to producing these pre- and post launch distributional forecasts” according to Smith Decision and Operations professor Xiaojia Guo and her co-authors.
Guo’s research, Bayesian Ensembles of Exponentially Smoothed Life-Cycle Forecasts, published in Manufacturing & Service Operations Management in October 2024, studies “the problem of forecasting an entire demand distribution for a new product before and after its launch.” Guo worked with Kenneth C. Lichtendahl Jr. at Google and Yael Grushka-Cockayne of the University of Virginia.
Guo said she and her co-authors were trying to develop a new method for product life cycle prediction. “I think, before our work, there were some well-established models for predicting product life cycles, but they were not tailored to new product categories that have emerged in recent years, such as fast fashion goods, online news topics, or things like YouTube videos. They have short life cycles, yet valuable insights can be drawn from similar past products,” she said. According to the research, “each candidate model in our ensemble is a life-cycle model fitted to the completed life cycle of a comparable product.”
Using a mathematical model that captures similarities between a new product and a past product, the model “can aggregate previous products’ life cycles so we can have a single line of the future product’s life cycle,” Guo said, adding that the model is designed so that this information from previous products can help to inform data before a new product is launched on the market.
Another advantage is that the model also provides flexibility because it can be adapted to changes in the product’s life cycle. This was part of the inspiration behind the study, as Guo said an MBA student the author’s team was working with at the time was looking for a model that could help to predict something that has an increasing or decreasing trend.
“It’s a very widely applied approach. It’s called exponential smoothing,” Guo explained, so they combined that method with a new marketing model for product lifecycle and innovation diffusion. So, not only does the model play a role in predictions before the launch, but data can be updated after the product is released, allowing for greater flexibility.
Guo said since industries also have a better way to store data, compared to 10 years ago, they can access more information and understand demand across time.
As products have evolved and changed, such as the demand for cloud computing and considering promotional tactics for products and their impact on product demand, Guo said there are more areas to explore and potentially expand with this model.
“People think the area is already developed by the market but the practice has evolved even faster,” Guo said. “So we have to catch up on that and maybe have a new model for these kinds of products.”
Read Guo’s recent work, “Bayesian Ensembles of Exponentially Smoothed Life-Cycle Forecasts” published in Manufacturing & Service Operations Management.
Media Contact
Greg Muraski
Media Relations Manager
301-405-5283
301-892-0973 Mobile
gmuraski@umd.edu
Get Smith Brain Trust Delivered To Your Inbox Every Week
Business moves fast in the 21st century. Stay one step ahead with bite-sized business insights from the Smith School's world-class faculty.