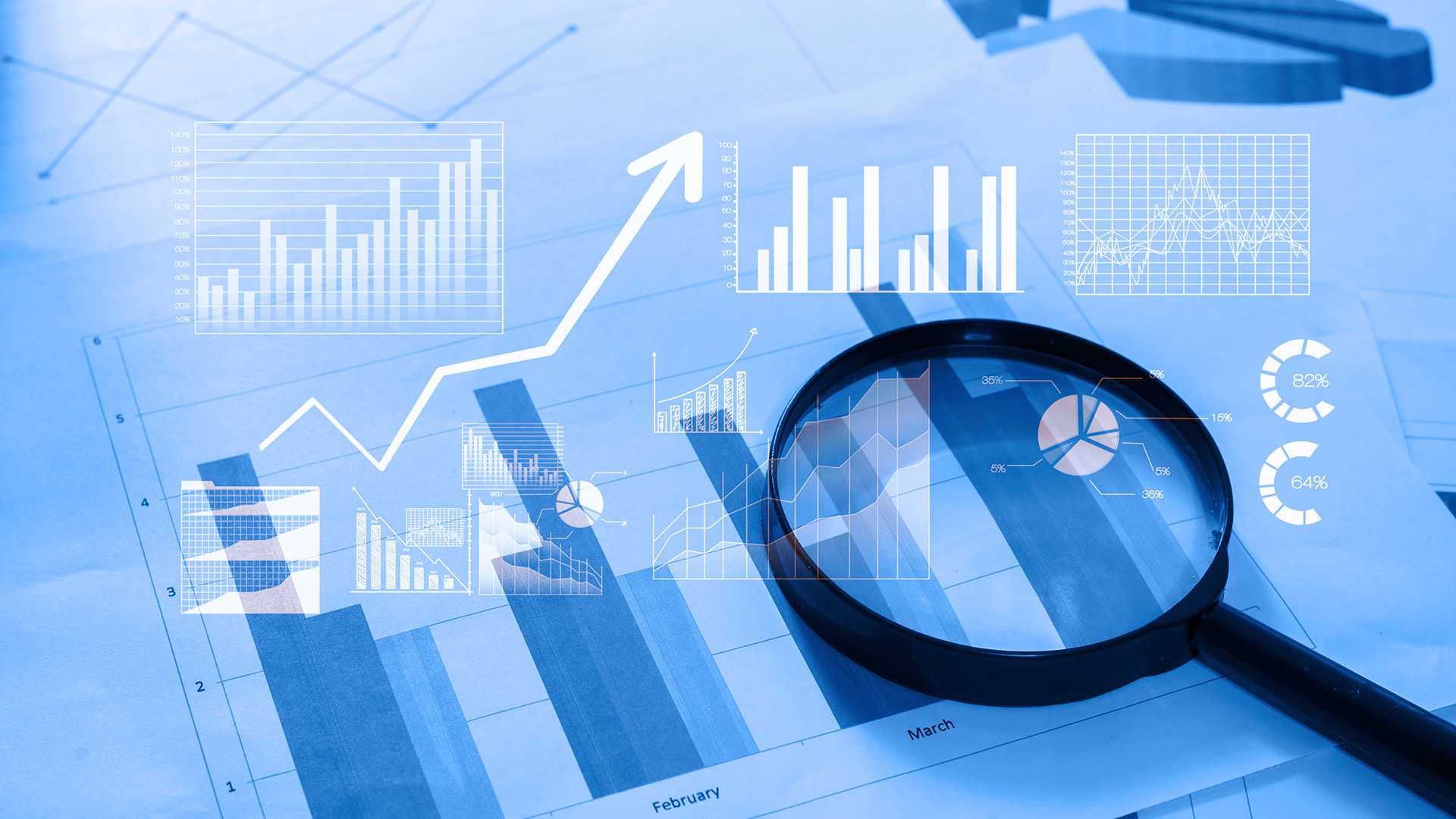
When it comes to investing, predicting stock return volatility is the name of the game. Traditional methods rely on stochastic models, but more recent models have turned to text-mining techniques. Now, research from Maryland Smith is taking those new methods even further.
Kunpeng Zhang, assistant professor of decision, operations and information technologies at the University of Maryland’s Robert H. Smith School of Business, joined by Yi Yang of Hong Kong University of Science and Technology, and Yangyang Fan of Hong Kong Polytechnic University, have created a new word-embedding method that seeks to improve volatility prediction.
Recent models are mostly comprised of dictionary-based matching algorithms using sets of manually constructed keywords that fail to track latent meaning within text. This new model proposed by the researchers addresses that shortcoming through its word-embedding method incorporating knowledge from a well-known finance-domain lexicon – the Loughran and McDonald word list.
The result? When compared with benchmarks across 10 years of annual reports from Russell 3000 firms, the newly proposed method significantly improves prediction error with a 28.4% reduction on average.
“Previous text analysis on firm disclosure heavily relies on bag-of-words models that miss a great deal of important semantics,” the researchers write. “Our research answers a call to apply machine-learning models to capture deeper semantics and latent context in business text.”
To make the model work, the researchers designed a new objective function that is able to learn word association under lexicon constraints, including must-link and cannot-link constraints. That design enables users to more efficiently and accurately analyze unstructured data like firm financial reports for the purposes of stock volatility prediction, the researchers write.
Regulatory agencies and other academic researchers may benefit greatly from this kind of tool, they write. Regulatory agencies can leverage it for forecasting market volatility by evaluating the discrepancy between a firm’s predicted and realized volatility. Academic researchers, on the other hand, may see it as “a general outline that uses domain-specific word embeddings as input for predicting a critical financial variable.”
But even investors will find the novel method to be useful, the researchers write. The financial-specific, word-embedding algorithm is available as open-source information that can be used by anyone to help analyze financial reports and assess financial risks.
“For investors, who often use traditional stochastic models, our method can serve as another tool for forecasting stock volatility. Volatility forecasting is of the utmost importance for institutions involved in option trading and portfolio management,” the researchers write. “It is crucial for them not only to know the current volatility level of their managed assets, but also to be able to estimate future volatility.”
Read More: “Analyzing Firm Reports for Volatility Prediction: A Knowledge-Driven Text-Embedding Approach” in the INFORMS Journal on Computing.
Media Contact
Greg Muraski
Media Relations Manager
301-405-5283
301-892-0973 Mobile
gmuraski@umd.edu
Get Smith Brain Trust Delivered To Your Inbox Every Week
Business moves fast in the 21st century. Stay one step ahead with bite-sized business insights from the Smith School's world-class faculty.