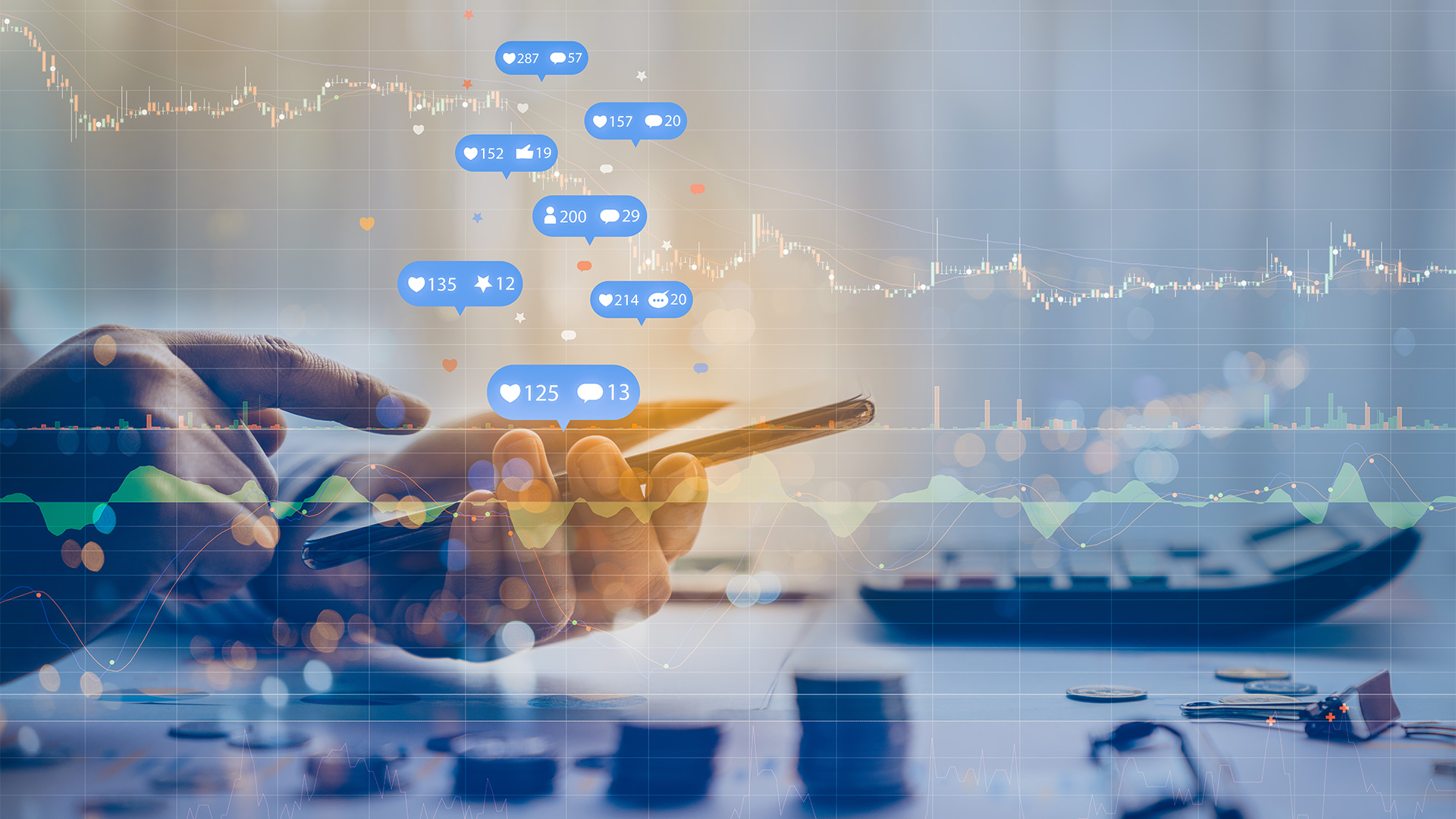
It’s clear that internet buzz and news affect stock prices – just look at the wild rides of 2021’s meme stocks like GameStop and AMC for proof. New research from Maryland Smith’s Prabhudev Konana creates a new methodology to translate just how news and internet chatter can impact stock prices. The research also recommends a trading strategy for taking advantage of all that chatter.
Konana, dean and professor of information systems, collaborated on the research, published in Information Systems Research, with co-authors Wuyue (Phoebe) Shangguan of Xiamen University in China, Alvin Chung Man Leung of City University of Hong Kong, Ashish Agarwal of the University of Texas at Austin, and Xi Chene of Zhejiang University in China.
They created a metric that makes it possible to actually measure just how much information (e.g., social media chatter) flows that can move stock prices. The metric takes into account the intensity of a stock’s attention online, which allows for more accurate forecasting of returns, say the researchers.
Konana and his co-authors adapted an existing network mapping tool to online information about public companies, using it to look at traditional media sources – including news stories and analyst reports – as well as social media posts and comments among investors.
The new metric significantly outperforms other existing measures in predicting the direction and magnitude of abnormal returns of stocks. Abnormal returns represent firm equity value beyond what is expected based on a set of market risk factors using asset pricing models, say the researchers. They are commonly used to predict stock performance.
The researchers say their model can be used to assemble a stock portfolio and develop a trading strategy that provides “significant and positive excess returns.” They say using the combined information that the model puts together is a much better way of predicting performance than looking at each information source separately because it takes into account social media attention instead of just traditional media attention.
To create the model, Konana and his co-authors gave each stock a score based on attention a stock receives which they call EAC. The higher the EAC, the more online information flow there was about the stock. They also look at the sentiment of the information – whether the mentions about a stock were positive or negative.
They put their model to the test, using it on data from the Chinese stock market from June 2015 to July 2016. They found adding EAC to a model makes it up to 32% more accurate in predicting abnormal returns. And social media predicted returns more accurately than traditional media, because even if the original mention of a stock came from an analyst or news story, it got a boost when it spread on social media.
Interestingly, meme stocks didn’t fit the effect because of the rapid spread of information about them that is quickly reflected in a stock’s price, say the researchers. For those stocks, higher levels of information flow bumped up their EAC but actually reduced their abnormal returns, by 4.44% for each 1% increase in EAC.
The researchers use their model to come up with a trading strategy to buy stocks with low EAC and positive sentiment, and short stocks with negative sentiments. With this strategy, they could capture higher excess returns by getting in on stocks before their gains picked up, with the start of positive chatter about them. They tested the strategy on Chinese stock market data in 2017 and showed it generated profits of up to 0.33% a week.
The researchers say investors could use their strategy by incorporating EAC values in automated algorithms. And, they say, their EAC model could be useful beyond stock markets. For example, companies could use it when deciding which new products to develop and to forecast consumer demand and sales.
Read the research, “Developing a Composite Measure to Represent Information Flows in Networks: Evidence from a Stock Market,” in Information Systems Research.
Media Contact
Greg Muraski
Media Relations Manager
301-405-5283
301-892-0973 Mobile
gmuraski@umd.edu
Get Smith Brain Trust Delivered To Your Inbox Every Week
Business moves fast in the 21st century. Stay one step ahead with bite-sized business insights from the Smith School's world-class faculty.